Text Analytics Key To Unlocking Big Data ValueText Analytics Key To Unlocking Big Data Value
Big data is set to transform business, but text analytics will play a huge role in that transformation.
July 11, 2013
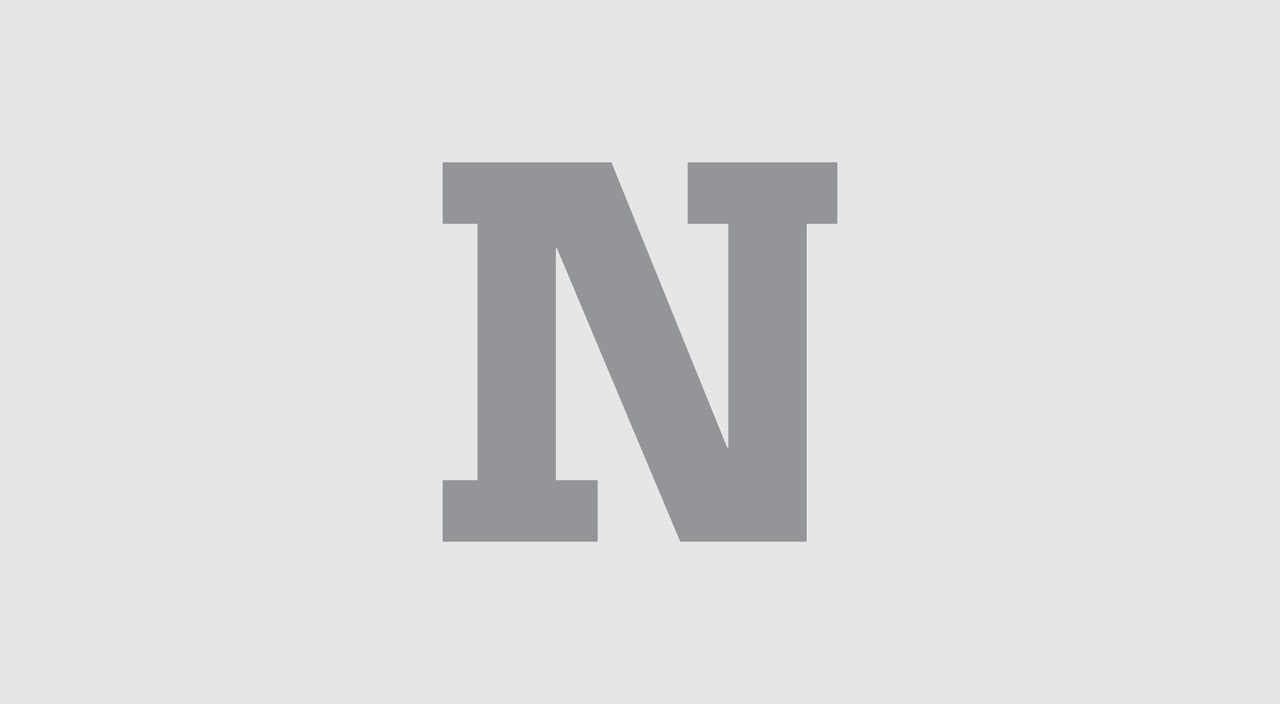
The talk these days is all about big data, but extracting insights that lead to value is the role of analytics--not just big data alone. And since a lot of that data is textual in nature, the responsibility for delivering value falls upon textual analytics. And that is a big deal.
I recently attended the Text and Social Media Analytics Summit in Cambridge, Mass. The conference highlighted the increasing importance of text analytics. Let me touch upon just a few of the ideas discussed at the meeting.
Good Analytics Crucial To Deriving Big Data Value
Gary King of Harvard University gave one of the most thought-provoking presentations, with the challenging title of “Big Data Is Not About the Data.” His thesis was that the value of big data actually lies in the analytics. To prove his point, he cited an examination of the solvency of the U.S. Social Security Administration (SSA). The SSA had used essentially the same statistical methods for 75 years, and overall SSA forecasts were inaccurate, inconsistent and overly optimistic. Through the use of customized analytics that King’s group at Harvard developed, forecasts using publicly available information showed that the SSA Trust needs over $1 trillion more than it thought.
[Read about Pivotal, the big data venture spun out of EMC, in "Pivotal Is About Big Data, Not Fighting Amazon."]
King said this type of analysis would also apply to the insurance industry and public health, among other areas. His argument on the value of analytics over data seems to be that the data was already available, but extracting value depended upon building analytical techniques that could unlock that value.
Although King makes a strong point, the answer is that both data and analytics are important. All the analytics in the world will be of no help if the data does not exist or you cannot access the data for use. Still, King’s thesis really speaks to the need for creativity in the use of analytics to take advantage of data.
Integrating Structured And Unstructured Data Concepts
Traditional analytics has tended to focus on structured data--that is, relational databases (such as doing analyses using traditional data warehouses). Much of big data tends to fall into the unstructured data category. (I distinguish between semi-structured and unstructured data, but I won’t push the difference here.) Unstructured data tends to respond to analytical techniques such as text analytics, rather than the analytics typically applied to SQL data. This has led to the thesis that the two are separate and distinct (as well as to the thesis that non-SQL techniques will dominate).
Ralph Winters of Emblem Health and other speakers at the conference vigorously disagreed with this point of view. In his presentation, “Practical Text Mining with SQL -- Using Relational Databases,” Winters clearly showed the value in mapping unstructured data to structured data with a full-text search that led to a weighted word matrix and other types of structured analyses. This could be used to spot churn or conduct a sentiment analysis. Tying a relational database to such things as a Hadoop connector, open source text mining tools and file interfaces can lead to increased analytical richness.
The whole field of text (and other) analytics continues to evolve. Integrating analytic concepts that have traditionally been applied with structured data along with techniques that have traditionally been applied to unstructured data shows great promise.
Text Analytics Has Many Practical Uses
Many examples were discussed during the summit, so I hesitate to focus on just one presentation, but Sergei Ananyan, CEO of Megaputer Intelligence, did a good job of discussing the business applications of text analytics.
In the 21st century, text analytics is taking advantage of machine learning, semantic analysis and deep linguistic parsing. All that can lead to useful applications, such as loan default analyses and sentiment analyses, according to Ananyan. One of the more important areas is medical diagnostics, where early diagnostics can eliminate common source of error. Another example is the use of text analytics in e-discovery, which is the examination of electronic information for evidence in a legal case.
Mesabi Musings
We have been subject to an application-driven software intelligence perspective of IT--where applications have dominated our consciousness as to where we derive value from IT--for most of our lives. So a data-driven software intelligence perspective such as big data, where value in IT is squeezed from the data itself, is not only unfamiliar and hard to comprehend but also a little uncomfortable. Yet the world of data-driven software intelligence is the world of text analytics and will transform our view of how to get value from the IT infrastructure.
Pay attention to what is happening as it will affect your business life more and more.
About the Author
You May Also Like