The Rise of DPUs: Revolutionizing App Performance and DeliveryThe Rise of DPUs: Revolutionizing App Performance and Delivery
In a future where DPUs are not only in data centers, every device from the edge to the core will be able to accelerate data tasks. As such, the real killer consumer use case for DPUs might be truly real-time applications.
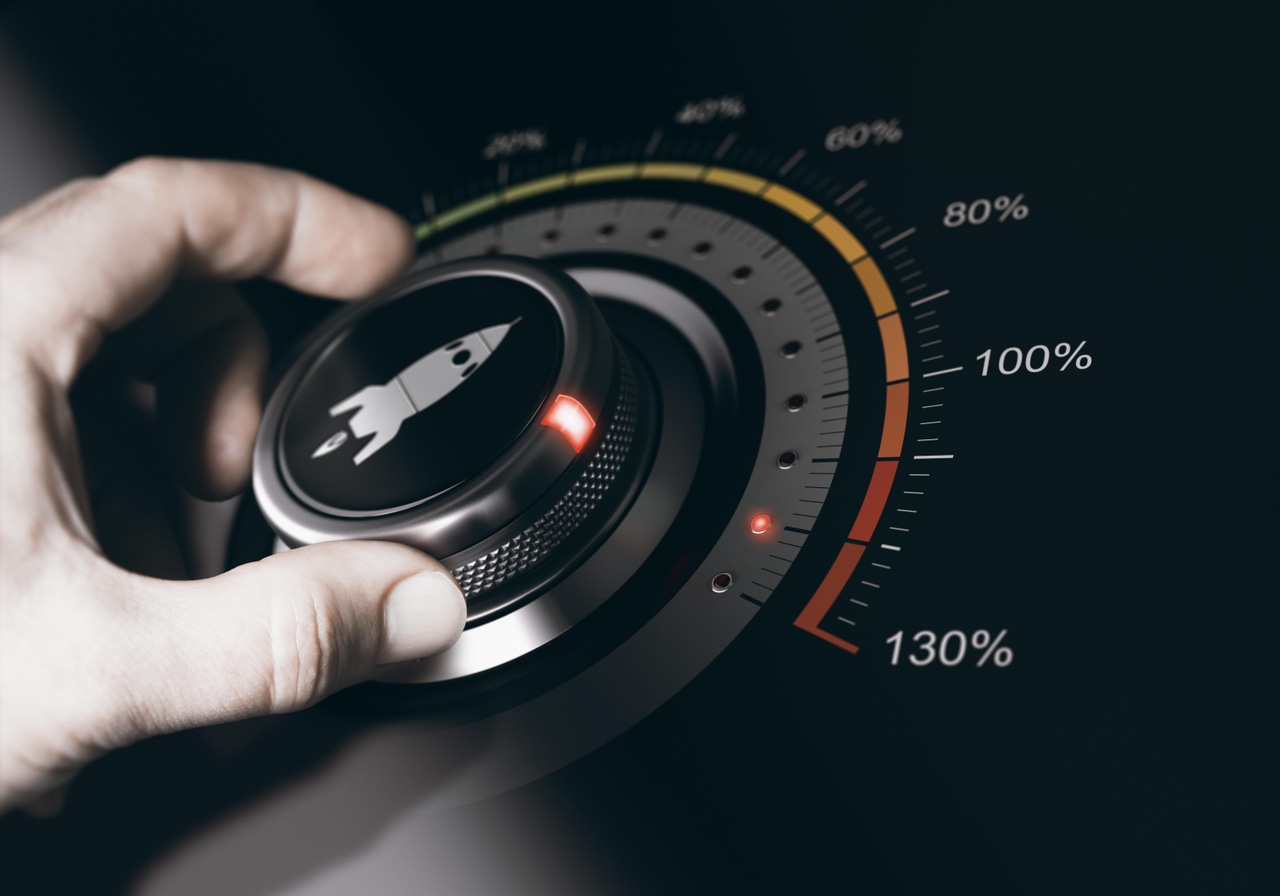
The OpenAI demo of GPT-40 broke new ground for AI applications. In one memorable section, two GPT-4o bots had a conversation and even sang together. This display was amazing, both that it was live and that the latency toleration for this to work without awkward pauses or interruptions is staggering. Of course, OpenAI and all the other big AI vendors have built AI-centric data centers. However, the secret of lightning-fast application response is not the marquee GPUs. Rather, a newer kid on the block, the DPU (data processing unit), is playing a critical role as a latency killer.
As AI workloads push the limits of application delivery and networking infrastructure for cloud giants and their customers, DPUs are poised to upend the traditional network stack. Soon, they'll be as ubiquitous in server rooms as CPUs and GPUs.
This shift promises to accelerate all applications, make them more secure, and make them more consistent. Ultimately, the DPU will spread to consumer devices where the need for speed is perhaps greatest. The upshot? The second half of the 2020s will see DPUs revolutionize app performance and delivery.
DPUs, the Hidden AI Accelerators
DPUs are specialized processors designed to offload and accelerate data-centric tasks, freeing up CPUs and GPUs to focus on their core strengths. DPUs generally have their own CPUs as well as high-speed networking connectivity, high-speed packet processing, multi-core processing, memory controllers, and other acceleration components. DPUs began to penetrate the data center in the early 2020s when AMD, Intel, and NVIDIA all announced the addition of DPUs to server chips to accelerate processing speeds and boost power.
DPUs are similar to Field Programmable Gate Arrays (FPGAs) and SmartNICs (network interface cards). A key difference is that DPUs carry significant compute power of their own and can be adapted for a wide variety of use cases. In contrast, FPGAs tend to be less powerful and SmartNICs focus on encryption and security.
Many companies today deploy DPUs as part of their product offerings. HPE Aruba uses DPUs for network acceleration, and Dell uses DPUs to improve performance on its servers. There is even a software-defined DPU designed for edge devices and unforgiving environments.
The emergence of ChatGPT and improvements in AI set off an arms race to train and build machine learning models, services, and applications. This made DPUs even more important because they can offload costs and reduce the amount of GPU power and time required to execute AI-centric tasks. With the price of GPUs remaining exceptionally high, both training AI models and running inferencing needed to respond to queries for AI applications remain prohibitively costly.
Increasingly, DPUs are taking on tasks like data pre-processing, model compression, and data movement and running them alongside GPU processes. For example, a DPU can handle the complex image decoding and resizing operations required for computer vision models, saving cycles on the GPU and increasing model training speed. DPUs also reduce power consumption on AI workloads, a hot-button topic for data center operators facing an AI energy crunch.
DPUs' ability to efficiently move massive AI datasets around the network is a critical advantage for real-time AI applications that require rapid processing of large amounts of data. DPUs can enhance security for AI models and data by providing hardware-level isolation and encryption and ensuring data privacy. As for server CPUs running in the same system or alongside a DPU, these new processors allow the traditional workhorses to focus on sequential logic-heavy computational tasks better suited to their architectures.
Fulfilling the Need for Application Delivery Speed
While useful at the data center, DPUs are deployed on edge devices like base stations for 5G cell phone networks. It’s only a matter of time before DPUs start showing up on laptops and in smartphones as these devices incorporate more and more memory and processor intensive AI applications such as asking an AI model to process real-time video feeds when you are trying to fix a leak under the sink.
But the real killer consumer use case for DPUs might be truly real-time applications. Round-tripping complicated requests to an AI service in the cloud-delivered via API can often take multiple seconds and feel slow. In a future state with autonomous cars, drone delivery systems, and autonomous surgical robots, where onboard decisions are being made in milliseconds, that lag won't just feel too slow -- it will be too slow with potentially serious consequences. The pressure for faster and faster app delivery will only increase, and that will increase pressure to roll out DPUs in more places.
In a future where DPUs are truly everywhere, every device from the edge to the core will be able to accelerate data tasks. This could dramatically cut latencies across the entire application delivery process. It will be especially critical for “real-time” applications that rely on AI systems processing live streams of information or images. That pressure for faster apps is ever-present. In the demonstration of GPT4o, the system corresponded effortlessly with a human. OpenAI has access to massive compute resources. Regardless, users everywhere will expect all applications to run faster. Fortunately, DPUs might be a key to meeting the new need for application speed.
Related articles:
About the Author
You May Also Like