3 Questions About Behavioral Analytics, Answered
Behavioral analytics can power faster, smarter threat detection and response. How can you make these tools work for you?
June 3, 2022
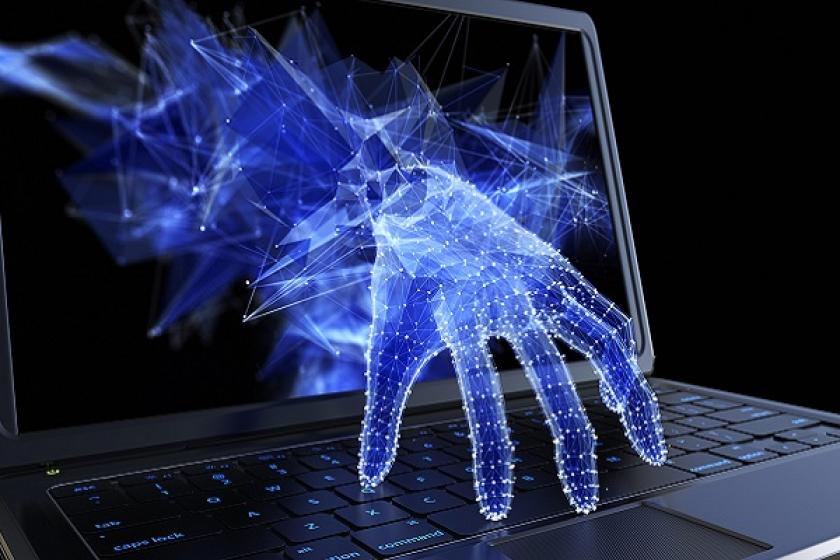
As cyberattacks continue to get more complex, SecOps teams are under increasing pressure to detect and remediate threats more quickly and effectively. To do so, many rely on security operations platforms with full threat detection, investigation, and response (TDIR) capabilities that utilize behavioral analytics. This helps reduce false positives, detects insider threats and zero-day attacks, and much more. But not all behavioral analytics are created equally. In this article, I’d like to explore some key areas of behavioral analytics by answering three questions that seem to keep popping up across the industry.
Before we dive into the questions, it’s important to define behavioral analytics (at a high level) and explain its role in threat detection. An effective behavioral analytics system will detect and adapt to both known and unknown threats. Behavioral analytics examines network, application, cloud, user, and device activity for behavior that is both unusual and high-risk. This requires machine learning (ML) models that baseline normal behavior, adapts to changes or anomalies, and homes in on actual threat versus legitimate anomalies (aka false positive).
In addition, conducting risk analysis involves determining the risk level of behaviors across all the telemetry and analytics performed. That requires gathering a large amount of contextual data (usually into a data lake), correlating, and linking that data to unique users and entities. Then a risk score is calculated based on that data and prioritized accordingly. This contextual information is the key to prioritizing which behaviors are risky or not, and includes relevant incident information such as events, network segments, assets or accounts involved (which can also be used by a security analyst to investigate and analyze a detected threat after the fact). Now on to the three questions.
Does it matter if my behavioral analytics use rule-based or trained Machine Learning models?
Absolutely yes. Although rule-based and trained ML behavioral analytics may seem similar on the surface, they function very differently. Rule-based detection models are often sold as ML, but they’re not. Rule-based detection is a form of AI that is essentially a flowchart that goes through a preset series of inputs regardless of context and generates an alert (or output) if predetermined criteria are met. Rule-based models often lack scalability, require constant updating by vendors to stay ahead of new threats and variants, and have fixed inputs and planned outcomes. This means they have no need to pull in a wide variety of data sources.
On the other hand, trained machine learning models (or “adaptable” machine learning systems), will self-train themselves and takes context into account to assess how risky or unusual a certain behavior is and adapt. For example, machine learning models can identify users accessing the network from unrecognized IP addresses, users downloading copious amounts of IP from sensitive document repositories not associated with their role, or server traffic from countries that the organization does not do business with.
What problems or roadblocks prevent organizations from using behavioral analytics successfully in threat detection programs?
One major problem that hinders many organizations is not gathering enough data to feed threat detection tools. If these tools don’t have a complete set of IT infrastructure and application data, then it takes much longer to determine if an attack is present. In fact, it forces security teams to manually figure out which data sets to pull on their own. Incomplete datasets also mean that threat detection isn’t as precise or contextual, which in turn means more false-positive alerts and more work for security operating center (SOC) analysts. Organizations often restrict their input data because they mistakenly believe that doing things like turning on NetFlow will slow down network performance. More commonly, organizations are leveraging a threat detection solution that charges based on the volume of data it ingests, so they are limiting inputs to keep costs from escalating out of control.
Another issue is data normalizations. Many rule-based threat detection solutions cannot ingest “unstructured” data from sources like proprietary business applications, Industrial Control Systems, IoT devices, or healthcare devices, because the data isn't in a format they recognize.
And finally, there’s the quality of the ML models that the solution is using. The more models a solution has, the more precise each one is. Models that can be tailored to unmask a specific attack campaign and adapt to new and emerging variants and attacks will be more accurate, and the solution will cover a wider range of security threats overall. A robust behavioral analytics solution should have hundreds or even thousands of ML models. Another challenge is that many solutions also have proprietary or “black-box” ML models that can’t be verified or customized. This also creates roadblocks because the analysts can’t validate that the models are accurate and working as intended, or let junior analysts examine the models to learn from them. In addition, proprietary models cannot be customized for specific environments or for an unknown attack. Open analytics, which does allow analysts to do this, is much more valuable.
How has behavioral analytics technology evolved in recent years and what changes are on the horizon?
Behavioral analytics technology has evolved significantly over the years with the implementation of true trained ML (moving past that traditional rule-based approach), which leverages supervised, unsupervised, and deep learning techniques. As malware has become more advanced and tactics like code obfuscation more common, rule-based systems have had a hard time adapting to the new malware landscape. While rule-based systems can potentially detect rudimentary attacks by more casual threat actors, targeted threat actors quickly adapt their tools and techniques to evade solutions overly focused on using this type of ML/AI.
The use of ML has allowed security teams to detect different kinds of threats and reduce costs in ways that could never be done before. Behavioral risk analytics has great potential to make threat detection more efficient and keep organizations safer, while reducing costs associated with a SOC. Building robust ML analytics that are drawn from as much input data as possible will be key to the success of this approach over the next several years as this technology becomes more standard in next-generation security systems.
Understanding the different types of behavioral analytics, as well as how they function within threat detection is key for businesses when implementing a threat detection system.
About the Author
You May Also Like