AI Workloads Spur Competition in Networking Chips
Cisco announced the Silicon One G200 and G202 networking chips that support AI / ML workloads. How are Broadcom, NVIDIA, and others keeping up?
July 13, 2023
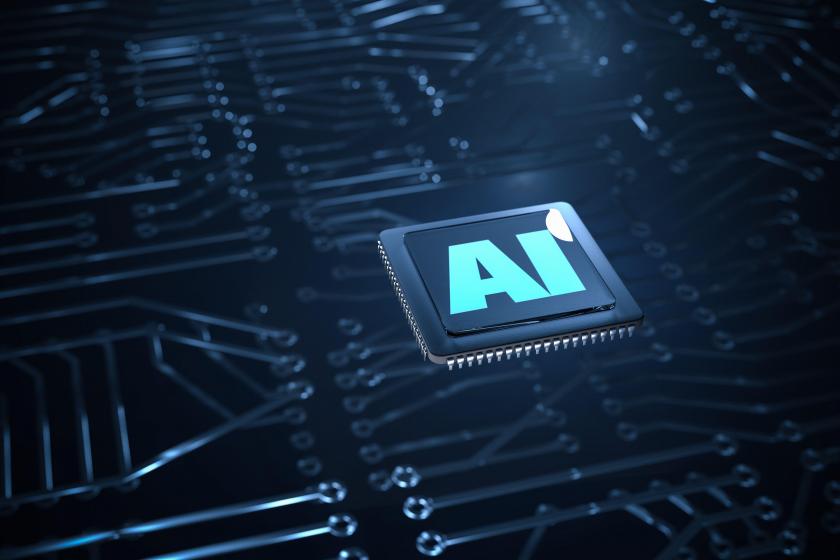
Networking vendors are competing in a tight market to produce networking chips that can handle artificial intelligence (AI) and machine learning (ML) workloads. Late last month, Cisco announced its Silicon One G200 and G202 ASICs, pitting it against offerings from Broadcom, NVIDIA, and Marvell.
A recent IDC forecast shows how companies plan to spend more on AI. The research firm predicts that global spending on AI will climb to $154 billion in 2023 and at least $300 billion by 2026. In addition, by 2027, almost 1 in 5 Ethernet switch ports that data centers purchase will be related to AI/ML and accelerated computing, according to a report by research firm 650 Group.
How Cisco networking chips improve workload time
Cisco says the Silicon One G200 and G202 ASICs carry out AI and ML tasks with 40% fewer switches at 51.2Tbps. They enable customers to build a 32K 400G GPUs AI/ML cluster on a two-layer network with 50% less optics and 33% less networking layers, according to the company. The G200 and G202 are the fourth generation of the company’s Silicon One chips, which are designed to offer unified routing and switching.
"Cisco provides a converged architecture that can be used across routing, switching, and AI/ML networks," Cisco fellow Rakesh Chopra told Network Computing.
The ultralow latency, high performance, and advanced load balancing allow the networking chips to handle AI/ML workloads, according to Chopra. In addition, enhanced Ethernet-based capabilities also make these workloads possible.
“Fully scheduled and enhanced Ethernet are ways to improve the performance of an Ethernet-based network and significantly reduce job completion time,” Chopra said. “With enhanced Ethernet, customers can reduce their job completion time by 1.57x, making their AI/ML jobs complete quicker and with less power.”
Cisco says the G200 and G202 also incorporate load balancing, better fault isolation, and a fully shared buffer, which allow a network to support optical performance for AI/ML workloads.
How chipmakers are tackling AI
Networking vendors are rolling out networking chips with higher bandwidth and radix, which are the number of devices in which they can connect to be able to carry out AI tasks, according to Chopra. They are also enabling GPUs to communicate without interference, eliminating bottlenecks for AI/ML workloads, he said.
“G200 and G202 can be used to train large language models (LLM) like ChatGPT and can also be used for inference of ChatGPT and other LLMs when customers interact with them,” Chopra said. “The Cisco Silicon One devices provide optimal connectivity between GPUs to enable ChatGPT and other advanced AI/ML models.”
The G200 and G202 offer visibility and telemetry features to be able to manage network loads and diagnose abnormal network behavior.
“If a packet drops, we can use it as a trigger to look back in time to see what happened leading up to [that event] and what happened after,” Chopra said. “This is very useful when trying to understand what is happening for microbursts because it uses hardware resolution rather than the traditional software resolution.”
Ron Westfall, senior analyst and research director at Futurum Research, said a network adding telemetry-assisted Ethernet to improve AI workload efficiency is like a car adding advanced software capabilities.
“It's really applying more brains to enabling the Ethernet network to perform more efficiently,” Westfall said.
An eye on the networking chips and devices market
Other networking devices designed to support AI/ML workloads include the Broadcom Tomahawk 5 and NVIDIA Spectrum-4, as well as the Marvell platform incorporating the Teralynx switch chip and Nova electro-optics platform. All of the switch chips in this category enable 51.2Tbps of bandwidth, Westfall noted.
“This is advancing Ethernet capabilities within the AI/ML cluster environments,” Westfall said. “They're all [aiming] to be a competitive alternative to InfiniBand technologies. As organizations look to use increasingly larger GPU clusters to obviously scale AI/Ml, we know that can be very demanding.”
The networking vendors that could come out ahead in the AI networking chip race are those that offer fewer switches, fewer optics, and a 256x radix-switch threshold, Westfall said.
“Cisco at least is making the competitive conversation more than about sheer performance and sheer scaling,” Westfall said. “It's about reducing the number of capabilities that have been used thus far to enable these AI/ML clusters to perform.”
That means using fewer direct-attach cables and fewer traditional optics, Westfall said.
Cisco could also add more flexibility for companies to build out their AI training clusters, which require more performance and processing power than AI inference models, according to Westfall.
“I think this is where Cisco can assert a fair degree of competitive differentiation because, obviously, Cisco is very much a juggernaut for Ethernet technology,” Westfall explained. “By boosting overall training and cluster efficiency, that is where Cisco can drive home that they can give customers a great deal more flexibility in how they approach building out their AI training clusters,” Westfall added.
How Broadcom and NVIDIA plan to keep up on Ethernet
Broadcom aims to keep pace with its Jericho3-AI high-performance fabric. Introduced in April, the fabric connects 32,000 GPUs.
Like the Cisco Silicon One chips, the Jericho3-AI offers load balancing and a high radix. Broadcom claims that the Jericho3-AI offers more than 10% shorter job completion times versus competing chips.
The NVIDIA Spectrum-4 Ethernet switch is also built for AI technologies such as ML and natural language processing. Like the Cisco chips, the NVIDIA Spectrum-4 offers features for telemetry.
Ethernet switches offer telemetry to allow for load balancing and spotting congestion on a network.
As network operators choose the chips for their AI/ML workloads, they will want to consider which ones can handle telemetry-assisted Ethernet, Westfall said. He also sees fully scheduled fabrics as important.
“This is something that I think we'll see more of, and that Cisco, through Silicon One, can enable more flexibility in allowing organizations to adopt telemetry-assisted Ethernet, as well as fully scheduled fabrics to basically take the scaling of AI training workloads and clusters to the next levels.”
What will make the chipsets successful with AI goes beyond bandwidth capabilities, according to Westfall.
“Even more important is which chipsets are going to be more effective, allowing customers to use less optics to basically become more able to reach these thresholds using fewer switches,” he said.
Related articles:
About the Author
You May Also Like